MCP vs. A2A: Navigating the Future of AI Agent Protocols
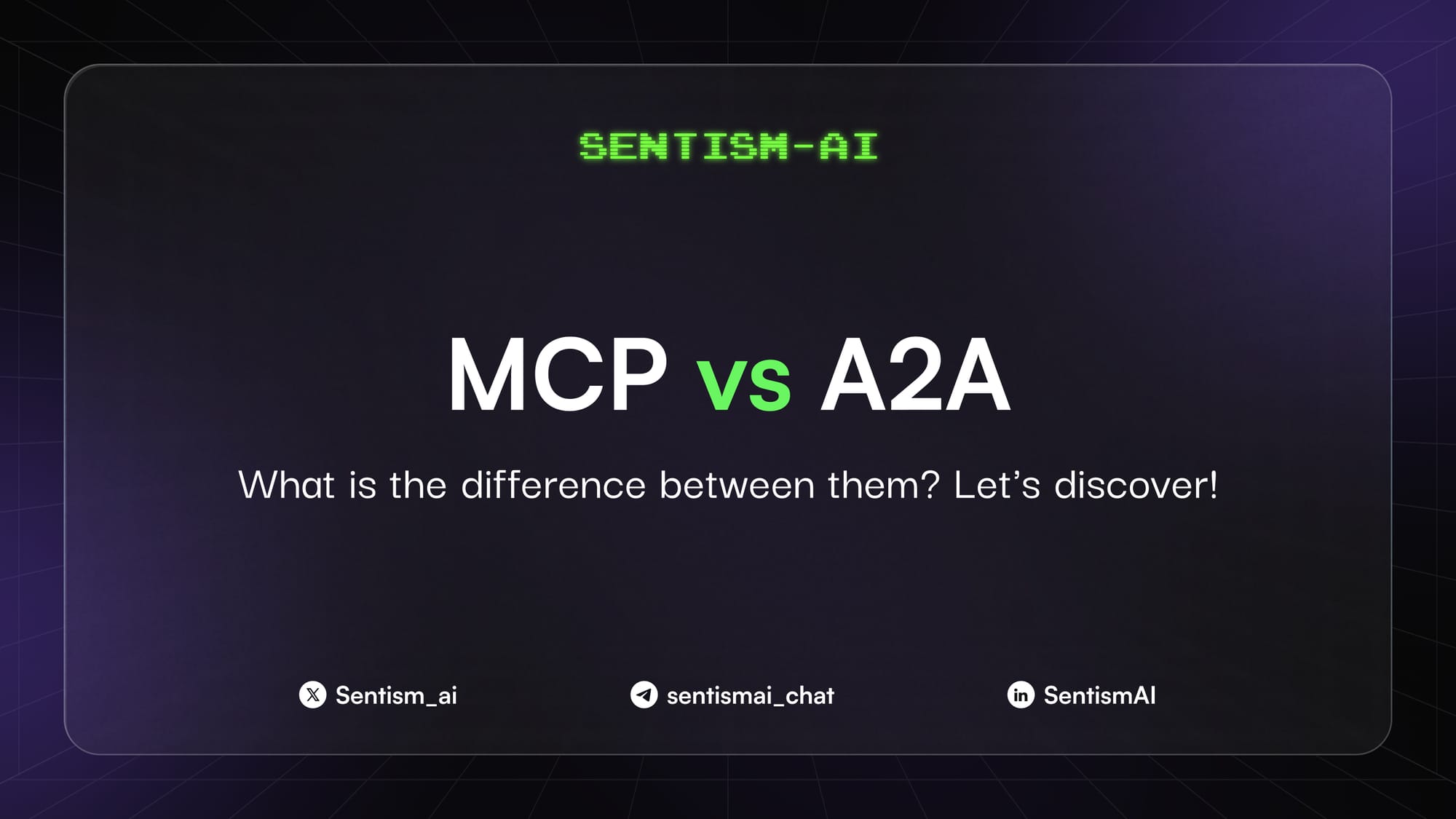
In the rapidly evolving landscape of AI, two protocols have recently emerged, promising to redefine how AI agents interact with data and each other: Anthropic's Model Context Protocol (MCP) and Google's Agent-to-Agent (A2A) protocol. While both aim to enhance the capabilities of AI systems, they approach the challenge from different angles.​
🔌 Model Context Protocol (MCP): Streamlining AI's Access to Tools and Data
Introduced by Anthropic, MCP is an open standard designed to simplify the integration between AI models and external data sources or tools. Think of MCP as a universal adapter, allowing AI applications to connect seamlessly with various resources without the need for bespoke integrations.​
Key Features:
- Standardized Integration: MCP provides a consistent method for AI systems to access and utilize external tools and datasets.​
- Modular Architecture: Its client-server model enables developers to build AI applications that can easily connect to MCP-compliant servers, facilitating scalability and flexibility.​
- Broad Adoption: Companies like Replit, Codeium, and Sourcegraph have embraced MCP to enhance their AI-driven platforms.​
By reducing the complexity of connecting AI models to diverse tools and data, MCP empowers developers to build more efficient and context-aware AI applications.​
Real-World Example:
Consider a software development environment where an AI-powered coding assistant needs to access a company's internal codebase, documentation, and issue tracker. By implementing MCP, the assistant can seamlessly retrieve relevant code snippets, understand the context of existing issues, and provide more accurate code suggestions, all without manual integration for each data source.
🔄 Agent-to-Agent (A2A): Facilitating Seamless AI Collaboration
Google's A2A protocol addresses a different aspect of AI integration: the communication between AI agents themselves. In complex systems where multiple AI agents operate concurrently, A2A provides a standardized framework for these agents to interact, share information, and coordinate actions.
Key Features:
- Inter-Agent Communication: A2A enables AI agents to exchange messages, negotiate tasks, and collaborate effectively, regardless of their underlying architectures.
- Enterprise Integration: With support from over 50 technology partners, including Salesforce, SAP, and ServiceNow, A2A is poised to become a cornerstone in enterprise AI deployments.​
- Built on Existing Standards: Utilizing protocols like HTTP and JSON-RPC, A2A ensures compatibility and ease of implementation across various platforms.​
By standardizing how AI agents communicate, A2A paves the way for more cohesive and efficient multi-agent systems.​
Real-World Example:
Imagine a customer support system where different AI agents handle various tasks: one manages customer inquiries, another processes returns, and a third updates inventory. Using A2A, these agents can communicate and coordinate to resolve a customer's issue efficiently. For instance, when a customer requests a return, the inquiry agent can notify the returns agent to initiate the process, which in turn informs the inventory agent to update stock levels—all through standardized A2A interactions.
🤝 Complementary Protocols or Competitive Standards?
While MCP and A2A serve distinct purposes - MCP focuses on connecting AI models to tools and data, whereas A2A emphasizes inter-agent communication—they are not mutually exclusive. In fact, they can be complementary in building robust AI ecosystems.​
Combined Use Case:
Consider a scenario in a manufacturing plant where AI agents monitor equipment, manage supply chains, and oversee quality control. An equipment-monitoring agent uses MCP to access real-time sensor data, while a supply chain agent uses MCP to retrieve inventory levels. Through A2A, these agents communicate: if the equipment-monitoring agent detects a potential failure, it can alert the supply chain agent to expedite the delivery of replacement parts, ensuring minimal downtime.
However, adopting and implementing these protocols will depend on the specific needs and architectures of AI systems. Developers and organizations must assess which protocol—or combination thereof—best aligns with their objectives.​
🚀 Looking Ahead
The introduction of MCP and A2A marks a significant step toward more interoperable and efficient AI systems. As the AI community continues to explore and adopt these protocols, we can anticipate a future where AI agents not only easily access diverse tools and data but also collaborate seamlessly to accomplish complex tasks.​
At Sentism, we're excited to see how these developments will shape the next generation of AI applications. Stay tuned as we delve deeper into the evolving world of AI protocols and their impact on intelligent systems.
For more insights into AI protocols and their applications, follow Sentism's blog and join the conversation on the future of AI integration.
Website | Whitepaper | Telegram | X - SentismAI | X - Zally | Telegram Mini App